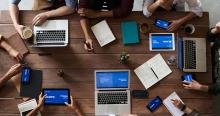
Business leaders are often searching for the best ways to make decisions in conditions of uncertainty. Simulation tools can be helpful in modeling and quantifying uncertainties – and simulation optimization can automate the search for better decisions in light of those uncertainties. But too often, typical simulation optimization tools don’t allow you to model the real choices that will help solve business challenges. There’s another level of simulation optimization that can help deliver optionality and recourse decisions, which helps your team “buy time” and flexibility to make better decisions today.
Let’s take a look at the benefits and limits of typical simulation optimization models, and show how to add optionality to your business decision-making.
How Simulation Optimization Benefits Your Business – and Falls Short
The idea of optimization is to help your business perform as well as possible: to maximize profits or minimize costs while working within constraints. But conventional optimization makes a big assumption: that all relevant input parameters are known with certainty.
Conventional optimization models ask business leaders to assume. For example, “We know exactly what commodity prices will be” or “we know exactly what interest rates will be” over the relevant time horizon. Often the attitude is that “the recent average price or rate is close enough.”
That’s often unrealistic, and may lead to quite negative business outcomes when prices or rates depart sharply from averages (as in a pandemic). So leading businesses turn to simulation optimization, where uncertain parameters such as commodity prices and interest rates are explicitly modeled, with probability distributions that reflect a range of possible values.
Simulation optimization works by exploring many combinations of values for the uncertain parameters, for every possible combination of the decision variable values. You can think of it as an “outer exploration loop” plus an “inner exploration loop.” On each pass through the “outer loop” the software tests a set of decision variable values, such as your spending decisions – one of 1,000s of possibilities. During that one pass, the inner loop runs 1,000 (or more) times, each time sampling a set of values for the uncertain variables, such as commodity prices or interest rates. Since there can easily be 1,000 x 1,000 or 1,000,000 trials, simulation optimization requires extra computing power.
With this in mind, what does it mean to “find the best decisions”? For any one decision (e.g., amounts to spend on different resources), simulation optimization explores 1,000 or more scenarios or outcomes. So we want a decision that performs well across all those outcomes. Depending on our business goals, “performs well” might mean “best average outcome,” “best worst-case outcome,” or “5% or less chance of doing worse than -$10,000”, and so on. Simulation optimization tools typically enable you to define such goals.
That all sounds great – and it is: Given the uncertainty and risk surrounding many if not most business decisions, simulation optimization offers very significant benefits over conventional optimization. This often justifies the extra effort of modeling uncertain variables, instead of making fixed “average case” assumptions.
But there’s something BIG missing from typical simulation optimization tools, that senior managers will quickly recognize when we point it out: The ability to model decisions that can be made on a “wait and see” basis – called recourse decision variables in the technical literature.
Two Types of Business Decisions: Here and Now vs. Wait and See
Let’s say you’re a farmer who needs to decide how much corn and wheat you want to plant. You will get a different price at harvest time for corn and for wheat. Your decision variables are how much corn and how much wheat to plant today. You know your budget constraints (how much land you have, the cost of seeds, etc.) and you understand something about the growth rates of each crop – which of course depends on rainfall, an uncertain variable.
A typical simulation optimization model will help you explicitly model how much rain you’ll get (low to high), and how much you can sell your corn and wheat for at harvest time. In agriculture, these uncertainties are a pretty big deal, and have a major impact on farmers’ business forecasting. So your simulation optimization model will surely include those uncertainties.
Here and Now Decisions: High Uncertainty
But . . . how much corn and how much wheat to plant is something you must decide “here and now,” before the growing season begins, before you know how much rainfall or what market prices you’ll get. If that’s your circumstance, you’re going to make a decision, and then the future will unfold.
Let’s say that corn is more sensitive to rainfall than wheat, and you’re highly uncertain about how much rainfall your crops will actually get. Even though you can get a higher price for corn, you decide to plant less corn and more wheat. It’s a way to hedge against the uncertainty of lower-than-expected rainfall to maximize your income.
What if there was a better way, instead of just planting your crops and hoping for rain? This is where recourse decisions, or “wait and see” decisions, can help. (The trouble is that most simulation optimization tools don’t support this, or even help you think about decisions different from “here and now.”)
Wait and See Decisions: Buying Optionality
What do farmers do in the real world of agriculture when there’s not enough rain? They irrigate. They bring in water. That’s called a “recourse decision.”
Here and now decisions are about making a decision with limited information and no extra time. Before the rainfall, before the future unfolds, you have to decide today – how much corn or wheat to plant.
Recourse decisions are “wait and see” decisions. With recourse decisions, you can decide what to do after the uncertainty is revealed. If your corn crops don’t get enough rain, you have recourse: you can bring in irrigation.
With recourse decisions, there is usually a cost involved. But the cost might be worth it. As a farmer irrigating crops, you can create a model (we call this a stochastic optimization model) that lets you see scenarios where you have to bring in X amount of water at a certain price. But this allows you to make better decisions “here and now” as well. A farmer can say, “I can bring in water, and when I run the numbers, even after paying the extra cost of irrigating that corn, I’m still better off planting more corn. I have a fallback option. I can still be profitable with corn, even if rainfall is light.”
Recourse decisions enable you to “plant more corn,” invest for the future, or conduct other equivalent operations in an atmosphere of uncertainty – and have better confidence in your business decision.
Recourse Decisions in Business: Unlocking Optionality
When you think about this for businesses, senior managers always talk about and are looking for “optionality.” They want recourse decisions. They want to buy themselves some flexibility so they can make better here-and-now decisions. The reason it’s called “optionality” is that business leaders will often happily pay a premium to buy themselves such options. There’s a whole academic literature on “real options,” which closely overlaps with recourse decisions – the difference is that stochastic optimization finds best or optimal values for the recourse decision variables.
Optionality empowers business leaders to make more profitable and less risky decisions. It helps solve operational challenges with better certainty, lower risks, and often with lower overall costs.
Businesses always need to manage costs, but sometimes it’s worth paying extra money in the short term to gain long-term flexibility. Business leaders will often ask, “Can we wait six months to make this decision? Can we get better clarity before we sign this contract? I’ll pay a premium to buy that extra time.” Industries like construction, insurance, and finance are all familiar with the value of optionality.
How Analytic Solver® Helps You Solve Business Challenges With Optionality
Recourse decisions are often underutilized, because too many simulation optimization tools lack the power to carry out the more complex stochastic optimization process required to use them. This type of “wait and see” thinking is totally missing from other tools supporting only basic simulation optimization like @RISK and Crystal Ball. With these other tools, you can’t even define a recourse decision or put it in your model.
Analytic Solver is the only fully supported simulation optimization tool for Excel that helps you model and solve for recourse decisions. Business leaders want to know what kind of decisions they can make on a delayed basis. Analytic Solver enables you to do that and have solution methods for both kinds of business decisions: here-and-now decisions, and wait-and-see (recourse) decisions.
With Analytic Solver, you can define and get multiple answers for multiple recourse decisions. For example, if you’re a farmer, and you want to see your options if rainfall is 10%, 30%, or 50%, of expected levels, Analytic Solver lets you see what to do for each scenario. It lets you see the scenarios in your simulation model for your chosen range of uncertainties, plug in what actually happened, and then obtain optimal recourse decision values, even if the real-world outcome wasn’t identical to one of the thousands of scenarios evaluated during the earlier optimization run.
Linde Solves Complex Distribution With Frontline
See how one of the world’s largest industrial gas and engineering companies used Analytic Solver® to account for uncertain demand while managing complex distribution across Brazil.