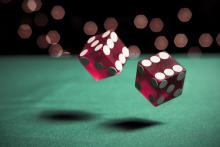
With today's tools, there's no reason to gamble on business decisions. Before making a decision involving uncertainty, managers and executives can – and should – insist that risks are quantified and explored.
While you can study your past data and business practices to learn from your successes and mistakes, a Monte Carlo simulation gives you a possible look into the future, and helps test your understanding of the situation today.
How Monte Carlo simulation works
The Monte Carlo method was invented by scientists working on the atomic bomb in the 1940s, who named it for the city in Monaco famed for its casinos and games of chance. Its core idea is to use random samples of parameters or inputs to explore the behavior of a complex process. To use this form of risk analysis you'll need numerical values related to the process, and basic analytical tools.
Let's say you want to plan for staffing a new customer service call center. You have estimates of fixed costs, variable costs per rep, call-handling times, and some past data on call volume. You start with a basic mathematical model that calculates costs based on your average handling time and expected call volume. This can be done easily in a spreadsheet such as Excel.
Next, you ask "which factors are uncertain?" – such as call-handling time, and call volume per hour in this example. For each one, you replace a fixed number with an "uncertain variable" that can take on a range of possible values. Then, you run a series of "Monte Carlo trials" (think of spreadsheet what-if scenarios) where you plug in randomly chosen values for each uncertain variable (within its own range).

Since you'll probably need thousands of trials to get a complete picture of possible outcomes, it certainly helps to have a computer do this for you. If you have a tool like Risk Solver, you can easily run Monte Carlo simulations in your Microsoft Excel spreadsheets, and visualize results quickly with charts and graphs.
Often, you'll get immediate insights from simply running a Monte Carlo simulation and viewing the results. Managers accustomed to thinking in terms of averages are often surprised by the range of possible outcomes the simulation reveals: just how bad – and how good – the results can be.
To make a decision, you'll need criteria for an acceptable outcome. Manufacturing.net said you should run Monte Carlo simulations with an expectation of how you want the final numbers to appear. For example, if the simulation shows that you're above break-even in 80 percent of the possible scenarios, you can probably continue forward. If outcomes are consistently below expectations, however, it's time to form a new plan.
Diverse functions of risk analytics
Atomic Heritage detailed how scientists working on The Manhattan Project used early computers to run risk analysis using random variables before putting ideas into practice. The scientists had a limited supply of uranium and wanted to explore every possible outcome before depleting resources.
You can use Monte Carlo simulation to preserve your own resources and get a look at investments before you leap, and you don't have to be an atomic physicist to understand it. Today, Monte Carlo simulations are run by businesses to plan investments, governing bodies to study traffic patterns, and hospitals to save lives. The Centers for Disease Control uses this form of risk analysis to account for a variety of factors that may affect public health in different outbreaks or hazardous material scenarios.
Getting an accurate range of possible values for each uncertain variable (a probability distribution) is a key step in creating a risk analysis model. Instead of simply using parameters dictated by market experts or other outside influences, you can use past data and distribution fitting to help you choose. Advisor Perspectives, a financial advice blog, suggested you can avoid Monte Carlo simulation problems by comparing the behavior of the distribution to your historical data.
In the modern world, it's not just "best practice" – it's essential to quantify your risks before making an important decision. And it's easier than ever before to do just that. Make it a part of your business practice to move from "thinking in terms of averages" to "thinking about the full range of outcomes," so you can make decisions with "both eyes open".